What we can do in post-production, and how easy it is to achieve, is about to change forever thanks to Generative AI.
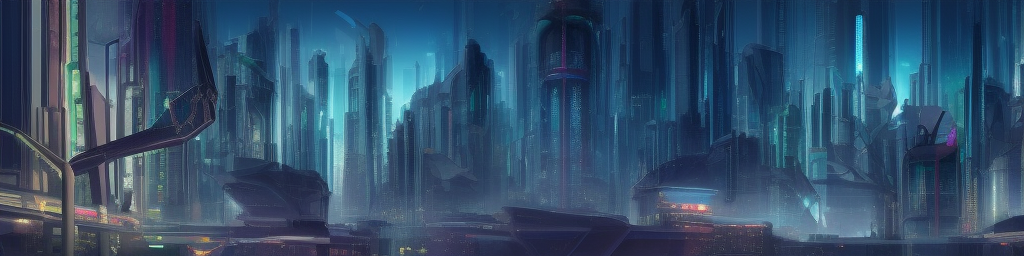
So, we experimented with this emerging technology to see what might be possible. Generative AI is the name for AI which has the power to create stuff. Whilst ChatGPT’s text function has been getting a lot of mainstream attention, the same generative capacity also exists for imagery. In fact, several AI platforms are now capable of creating entirely original images based on nothing more than a few simple prompts. So, we experimented with this emerging technology to see what might be possible.
The visual revolution
It’s all thanks to video games. Yep, that’s right. For all the fanfare that ‘deep learning’ in artificial intelligence is getting right now, the concept of neural networks in computing was first proposed in 1944 by Warren McCullough and Walter Pitts, two University of Chicago researchers who later moved to MIT. But it took video games, and the increasingly powerful Graphics Processing Units (GPU) that they required, to finally transform their abstract principles into today’s pretty awe-inspiring reality.
Thanks to the deep learning revolution, we now have generative artificial intelligence which is able to recognise and understand the complex mix of visual elements and styles which underpin the imagery we see every day. And off the back of this understanding, advanced algorithms have been developed which now allow AI to generate unique images based on specific inputs or parameters.
The possibilities that this presents for the worlds of art, design, advertising and entertainment are almost endless. But in this blog, we want to turn our attention to the potential for generative AI to transform video post-production; an area that is still very much in its infancy.
Why incorporating Generative AI into video post-production isn’t a slam dunk
Whilst we were excited to see what Generative AI could do with a simple test video, we knew there would be challenges:
Greater Complexity: After all, there’s a big difference between generating a single image, and generating 24 frames a second…
Computing Power: Videos need more computing power and storage capacity, which can make the AI generation process far less efficient.
Visual Continuity: Videos need to maintain continuity between frames, so that objects and scenes move or change smoothly, rather than jumping between different styles. This gradual visual evolution is a challenge for generative models.
Quality: As AI learning and prompting is still an on-going science, ensuring that a video maintains a consistently high resolution and quality, without any visual inconsistencies, isn’t easy.
But, given the pace at which AI has advanced so far, it’s highly likely that these problems will gradually be overcome. So, we got to work…
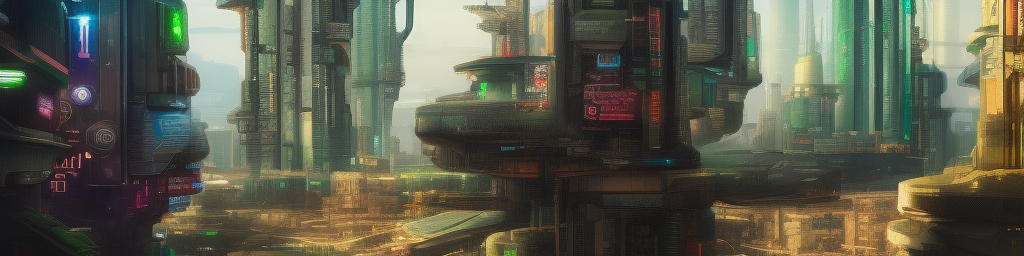
Our experiments
From the wealth of options out there, we chose Stable Diffusion for our test. That’s because, unlike DALL-E or Midjourney, it doesn’t impose artificial limitations on the images generated and its code is also open-source. That makes it a dream for developers, as it effectively allows us to look under the hood and not only better understand how the system operates (so we can work out how to get the best out of it) but also to design customized tools for greater flexibility.
We started by filming a simple video of one of our colleagues raising his arms. Then, we broke the video down into its component frames and fed this into Stable Diffusion as individual images.
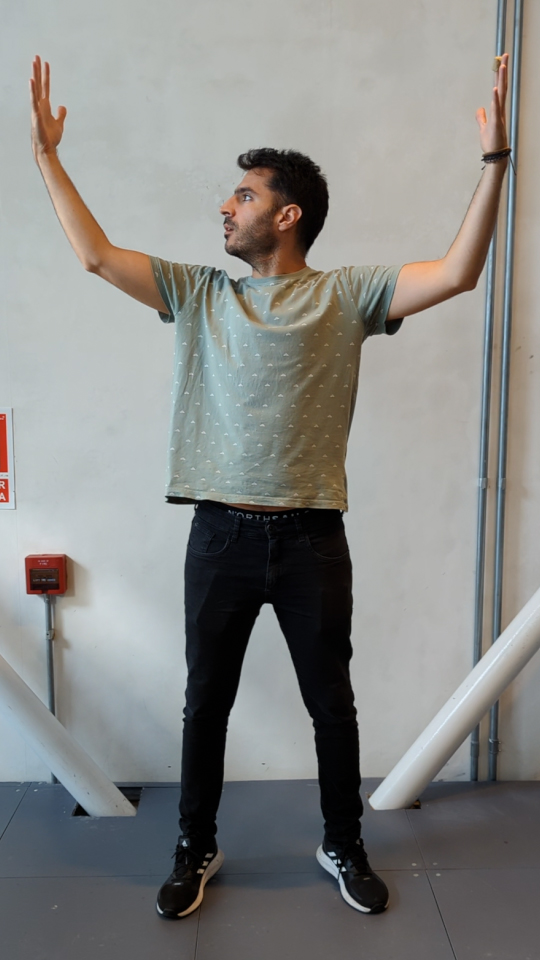
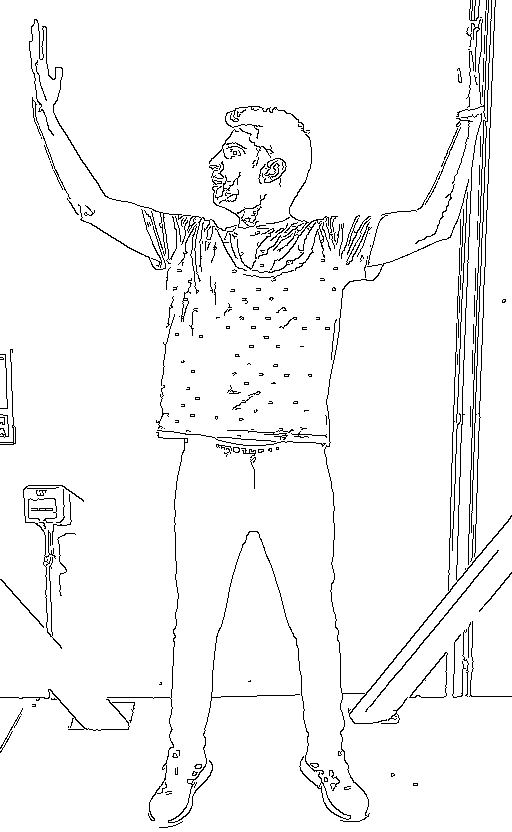
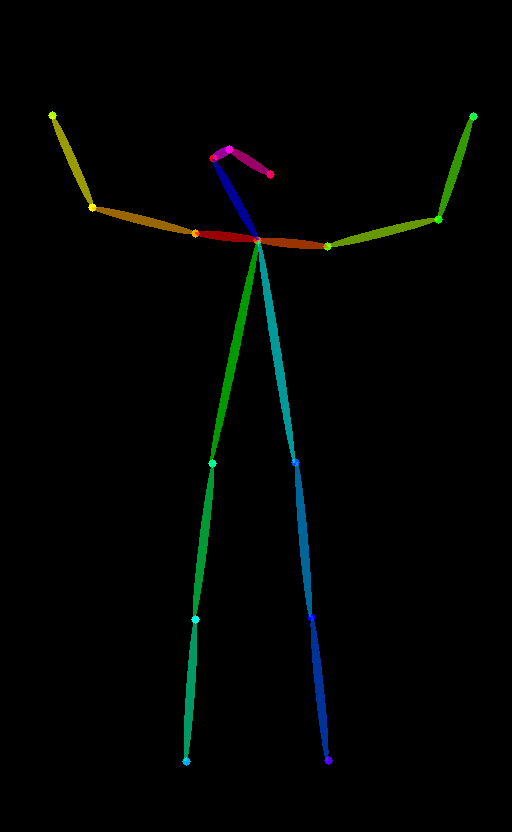
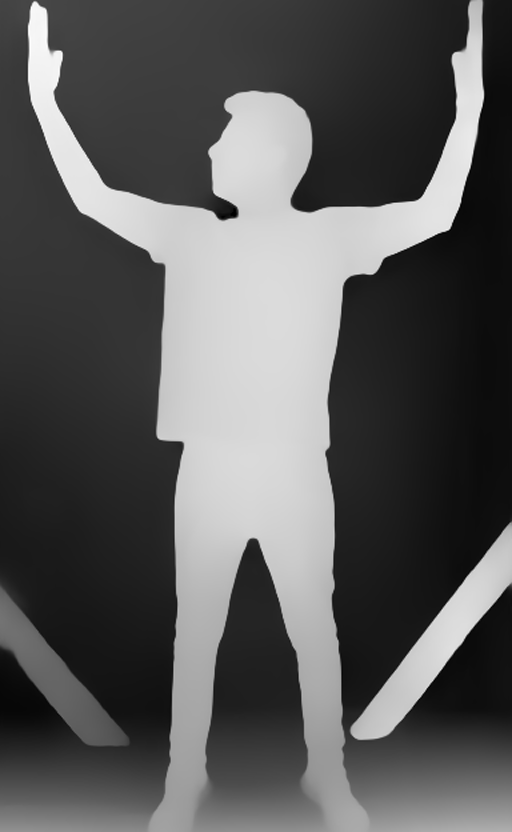
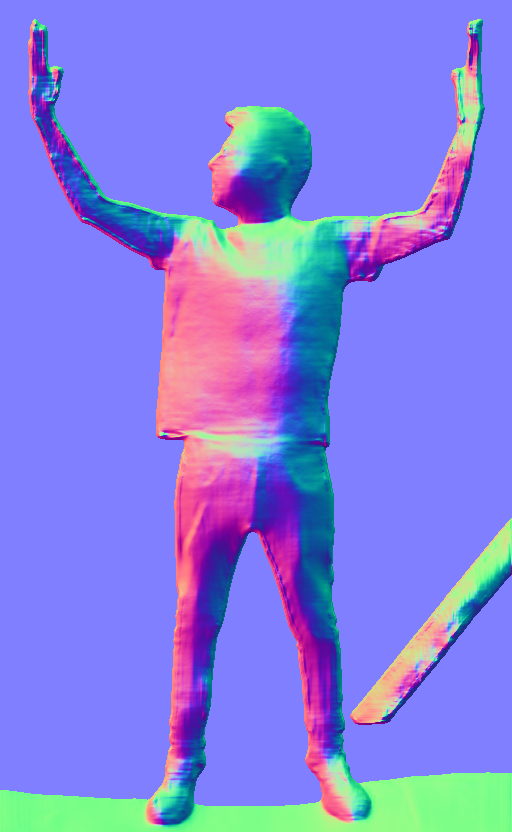
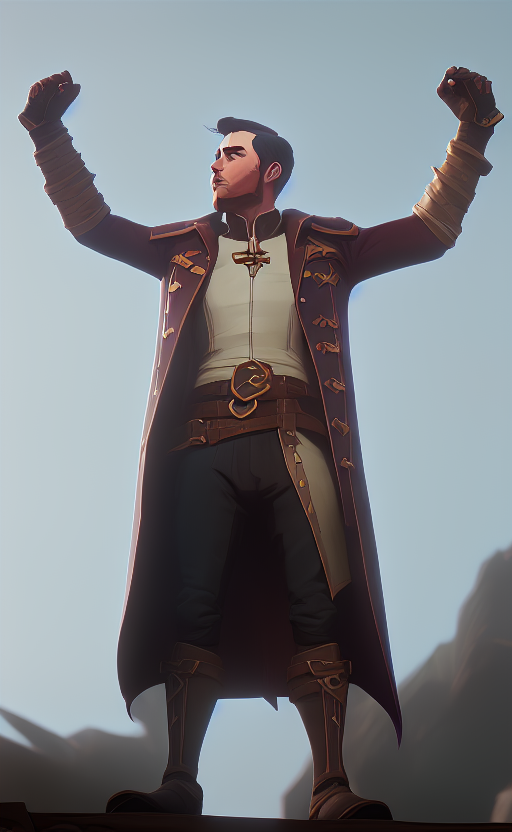
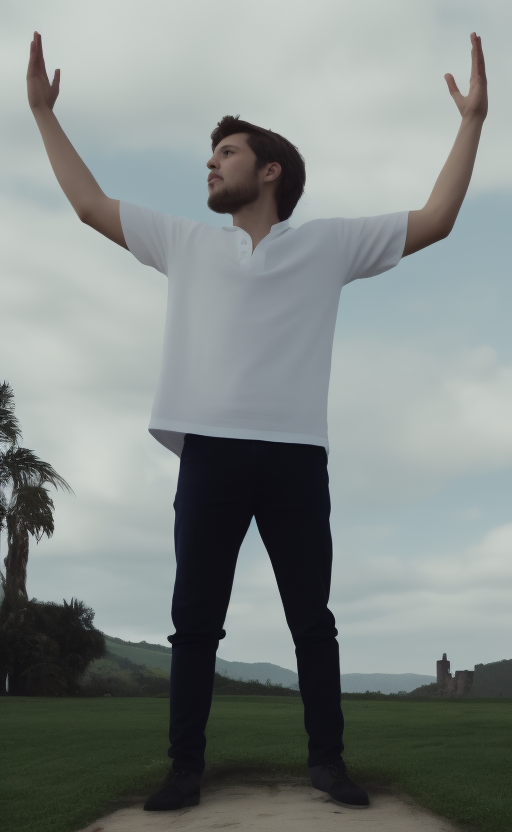
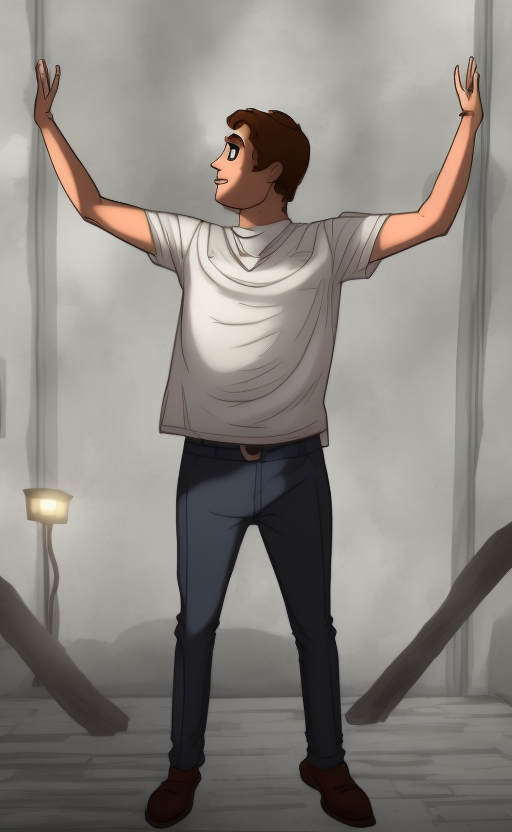
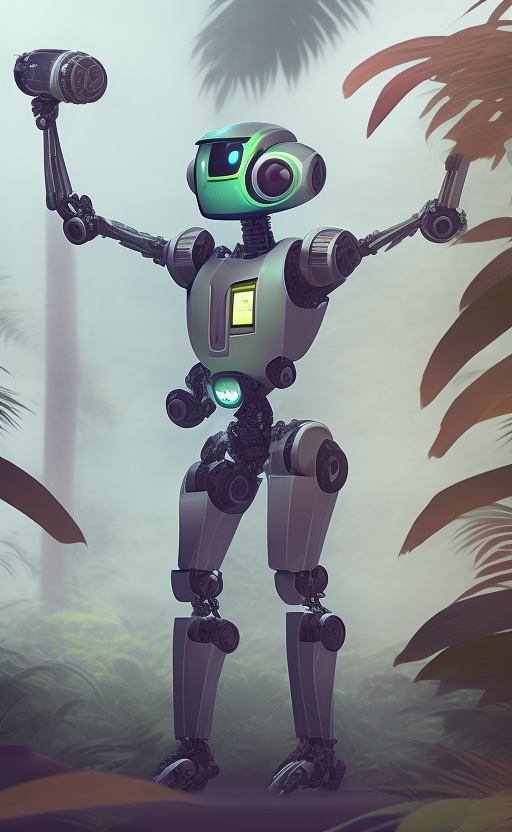
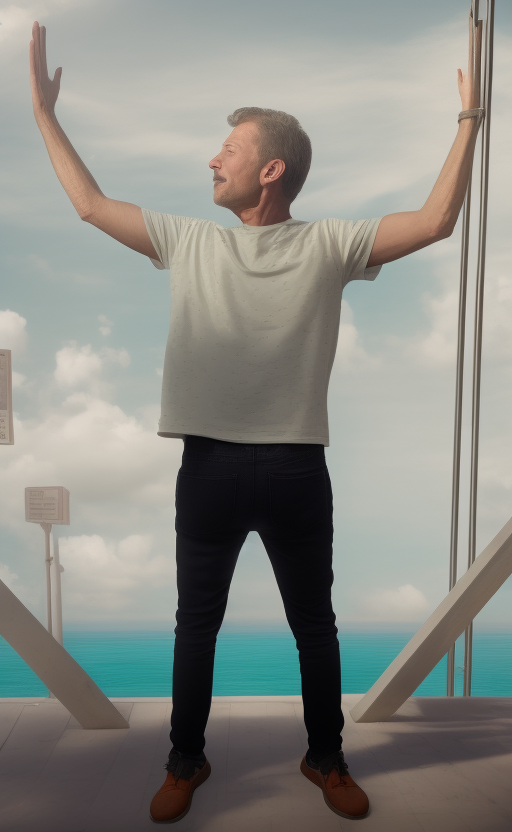
Stable Diffusion also comes with an extension called ControlNet which allows you to add extra input conditions to your image prompts. This addresses the problem of spatial consistency (one of the issues we flagged above) by essentially telling the AI model which parts of the image to keep. You can see the results in these first two videos.
Things were now getting very interesting. So, we decided to push the technology even further by combining our initial Stable Diffusion tests with another extension called EbSynth (a tool which allows you to animate existing footage). This allowed us to refine key frames individually, which generated an even smoother transition between frames whilst maintaining the original movement. You can see the difference this makes in terms of fluidity if you compare the first three portraits, all of which were produced using EbSynth, with the last portrait on the far right, which was not.
Finally, we used Low Rank Adaptation (known as LoRA) to train an AI model of our subject’s face and then entered this model into Stable Diffusion. As you can see in the example video, this created an extremely consistent result where the core facial features of our subject are maintained across the variety of different outputs.
What’s next?
Whilst all of this is impressive (and already lightyears ahead of what was possible a few years ago) it’s also clear that we’ve barely scratched the surface of generative’s AI potential. In fact, new apps are launching almost every week at the moment which push the boundaries yet further.
That’s why here at Labs we’re committed to trialing the latest tech and staying on top of each new iteration of AI development, because when we look at these new products, we’ve convinced that we’re watching the future of creativity being born.